Berggruen Seminar Series: AI – Ethical Challenges in Precision Medicine
As part of the Berggruen Seminar Series, Director of the Stanford Center for Biomedical Ethics Dr. David Magnus was invited to speak at Peking University on “Ethical Challenges in Precision Medicine.” Magnus is a Thomas A. Raffin Professor of Medicine and Biomedical Ethics, Professor of Pediatrics and Medicine at Stanford University, and Editor-In-Chief of the American Journal of Bioethics.
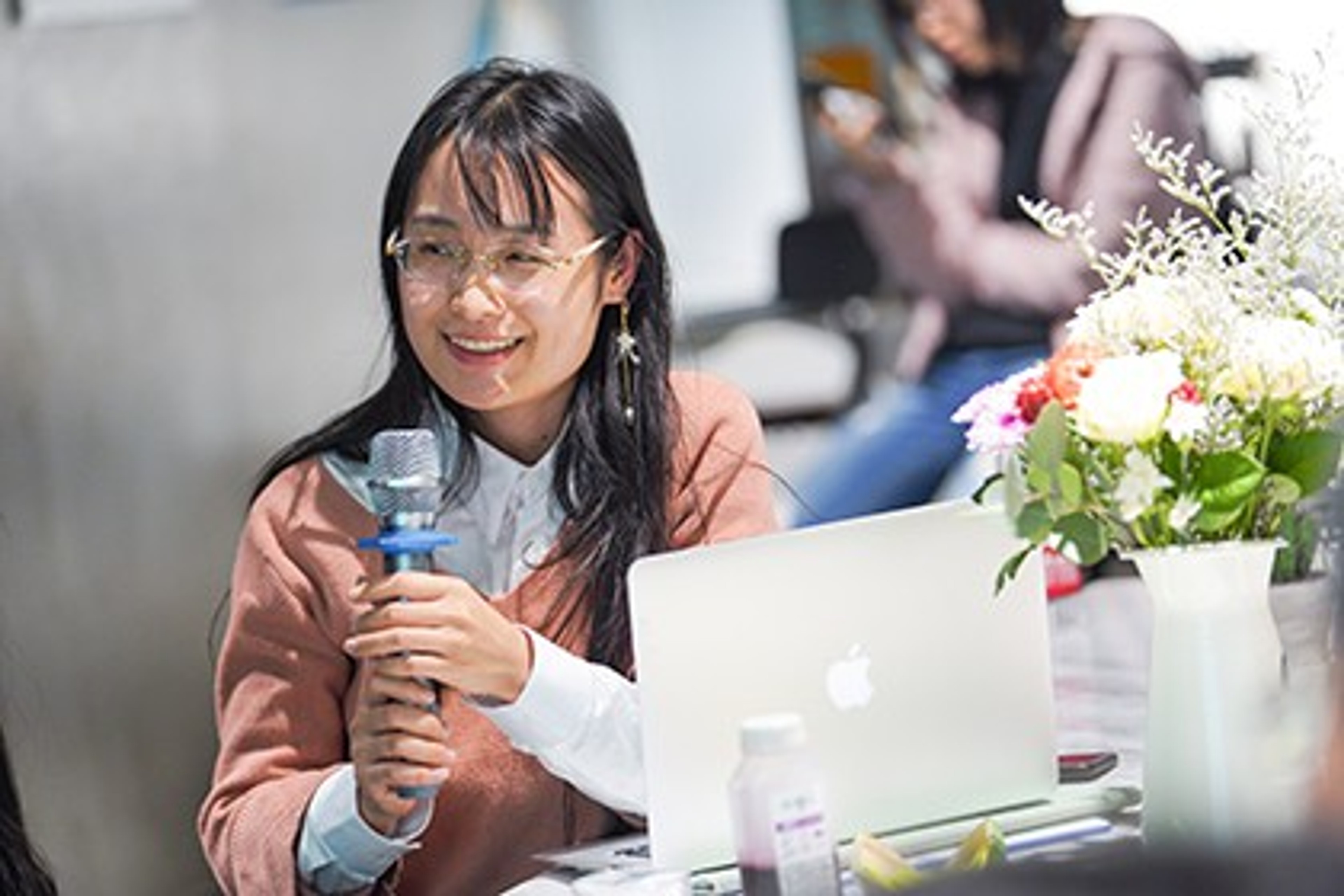
Magnus began the seminar by highlighting the need for precision medicine. Following current practice, physicians can only take a limited number of variables into account. The data used by physicians is often based on sporadic encounters with individuals within a specific healthcare system rather than a full range of available data. Moreover, Magnus pointed to the physician bias, wherein a physician’s account is strongly influenced by recent patient experiences.
Taking all these factors into account, computational tools such as machine learning would be required to fully comprehend and analyze the data. Magnus continued his talk by elaborating on the four major challenges in precision medicine (excluding privacy and security). The first challenge is the values embedded in the algorithm design process: If algorithms are developed by third-party vendors and sold back to healthcare systems, these algorithms can value different outcomes than those of patients or their physicians. A second major challenge is bias and limitation in the data. Here, Dr. Magnus pointed to the 2011 “Genomics for the world” research conducted by Bustamante, Burchard, and De La Vega, which showed that in the majority of genome-wide association studies, 96% of data samples are from people of European descent. Dr. Magnus accordingly questioned whether AI systems will be able to accurately make predictions if some populations are systemically underrepresented in the data fed to the algorithm.
Data arguably can lead to self-fulfilling prophecies in which biases wind up being absorbed through machine learning. Here, Magnus referenced his 2009 study on neurodevelopmental delay in pediatric solid organ transplant listing decisions, which analyzed the percentage of programs reporting how often neural developmental delay is a factor in transplant decision making. The study found that 44% of the programs always or usually take developmental delay into account when deciding whether to list somebody, while 39% rarely or never take it into account. This means that if machine learning is receiving information from an institution that does not list children for transplantation if they are developmentally delayed, the algorithm will see them as negative outcomes, thus reifying and reinforcing an existing bias.
Accurate and unbiased data also creates a significant dilemma. Zip codes are a widely used predictor of lifespan and life expectancy, as they encapsulate the fact that due to racial and ethnic disparities and indicators such as the Health-Wealth Gradient, both race and socioeconomic status are associated with significant levels of “excess death.” Machine learning is blind to consequence. Therefore, any algorithm that learns from data based on the reality that people are more likely to die earlier and have worse medical outcomes as a result of social inequalities will result in an algorithmic outcome that considers more affluent and therefore, statistically, more Caucasian people over others within the context of the United States.
We thus face a difficult dilemma in the algorithm design process, where the decision is either made to focus on the algorithm rather than the algorithmic outcome, arguably making the algorithm less predictive in terms of its outcome accuracy, or to focus on the algorithmic outcomes and predictions themselves, potentially reinforcing and reifying the social inequalities behind these problems.
Magnus raised this point to illustrate the difficult value choices that must be made concerning the algorithm design and voiced the concern that healthcare systems and clinicians might not even be aware of the data limitations or value biases in the algorithms, or in the data informing these algorithms.
For precision medicine to be effective for everyone, the data samples fed to the algorithms must have fair representation? and include underrepresented populations.
A first step in solving this issue is understanding the recruitment obstacles for data collection in scientific studies. Magnus referred here to the VALUES study (Patient Values and Attitudes about a Library of Knowledge: Uses of EHRs and Samples in Research), which aims to “assess and compare patient attitudes toward the use and governance of clinical data and samples in racially and ethnically diverse patient populations” and to “identify factors associated with attitude and preferences toward clinical data and sample use, sharing, and governance.” The study indicated that the choice of metaphorical terms requires careful consideration to generate the trust needed, especially among populations underrepresented in biobank-related research, to collect representative data samples.
The research participants showed a strong negative reaction to the commercial connotations of the term “bank” in biobank, suggesting there is a need to find a more appropriate metaphor. The research considered alternatives such as “Library of Medical Information” to be more representative of the key characteristics of a research “biobank.”
Magnus’ research highlights the importance of engagement and of recognizing cultural differences in building ties and relationships with communities in order to collect representative data samples needed to improve future medical research in precision medicine. He pointed to the four “needs” to prevent the misuse of algorithms: (1) the need for as much transparency as possible in algorithm design; (2) the need for close collaboration between clinicians and engineers as design takes place; (3) the need for awareness of values and interests in algorithm design; and (4) the need to reduce disparities in data.
The main takeaway from Magnus’ lecture is that an algorithm is only as good as the data it learns from. This realization prompts us to question: Can we realistically expect perfection from an algorithm that is fed with all our imperfections?